The Vital Responsibilities of Data Stewards: An In-Depth Analysis
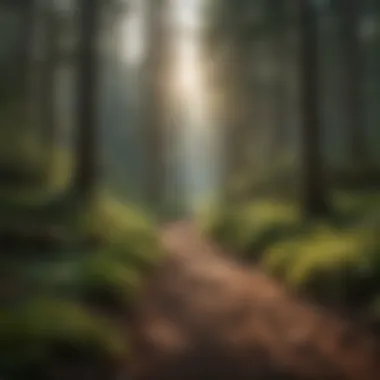
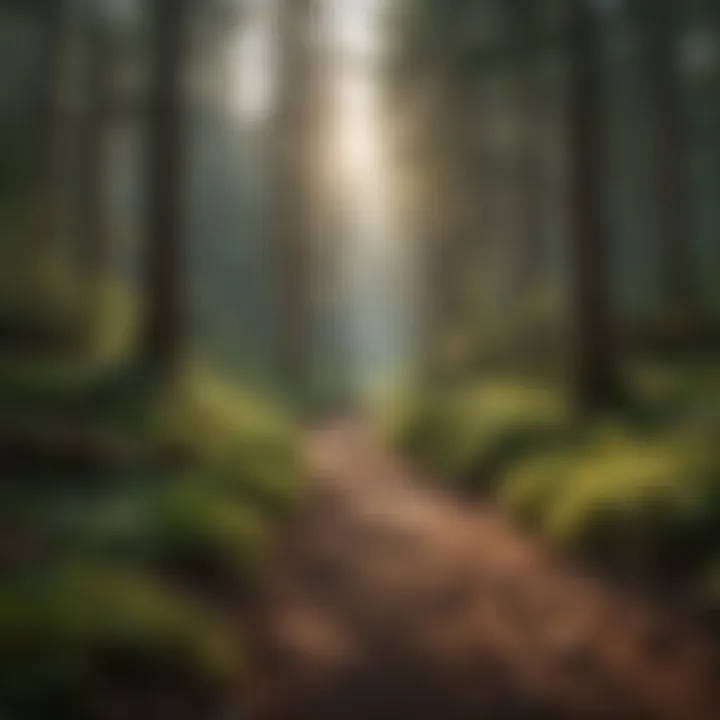
Management and Preservation of Evergreen Forests
Evergreen forests in the American landscape hold immense historical significance and are vital for biodiversity conservation. Native practices have traditionally contributed to the sustainable management of these forests, serving as a beacon for modern conservation efforts. The unique characteristics and resilience of evergreen trees underscore their value in preserving ecosystems and habitats that support a wide array of flora and fauna.
Ongoing research studies provide crucial insights into the biodiversity of evergreen forests and the sustainable management practices necessary to ensure their longevity. By staying informed about the latest findings and leveraging data-driven approaches, forest management professionals can develop comprehensive conservation strategies that encompass ecological preservation, species protection, and habitat restoration. The synergy between historical practices and contemporary research forms the foundation for successful conservation initiatives that safeguard American evergreen landscapes.
Initiatives aimed at the preservation of evergreen forests serve as beacons of hope amidst the challenges posed by climate change and deforestation. By spotlighting these conservation efforts, stakeholders can draw inspiration from successful endeavors and collaborate on innovative solutions to protect and nurture these precious ecosystems. The showcase of conservation triumphs not only highlights the resilience of evergreen forests but also emphasizes the collective responsibility to ensure their sustainability for future generations.
Introduction
In this article focusing on the comprehensive guide to The Responsibilities of a Data Steward, it is vital to understand the pivotal role data stewards play in organizations. Data stewards are the custodians entrusted with managing and safeguarding critical data assets. Their responsibilities extend from ensuring data quality to implementing governance policies, ultimately maintaining data integrity and enabling well-informed decision-making.
Understanding the Role of a Data Steward
Definition of a Data Steward
The Definition of a Data Steward encompasses the core concept of overseeing and protecting data within an organization. A Data Steward is responsible for defining data standards, ensuring data quality, and promoting data governance practices. This role is crucial for maintaining data accuracy and consistency across systems. The key characteristic of a Data Steward lies in their ability to bridge technical and business aspects, ensuring data aligns with organizational objectives.
Importance of Data Stewardship
The Importance of Data Stewardship cannot be overstated as it lies at the heart of efficient data management. Data Stewardship ensures that data remains trustworthy, accessible, and secure. By establishing data stewardship practices, organizations can enhance data quality, streamline data processes, and mitigate risks associated with erroneous data. This role is essential for fostering a data-driven culture within an organization.
Evolution of Data Stewardship
The Evolution of Data Stewardship reflects the changing landscape of data management practices. Over time, Data Stewardship has evolved from a primarily technical role to a more strategic and collaborative function. Modern Data Stewards are expected to possess not only technical proficiency but also excellent communication and problem-solving skills. This evolution marks a shift towards a more holistic approach to data governance, emphasizing the integration of technological advancements with organizational needs.
Significance of Data Governance
Key Objectives of Data Governance
The Key Objectives of Data Governance revolve around establishing clear guidelines and processes for managing data assets. Data Governance aims to ensure data consistency, compliance, and usability across the organization. By defining data governance objectives, organizations can enhance decision-making processes, regulatory compliance, and data integrity.
Integration with Data Stewardship
The Integration with Data Stewardship is crucial for aligning data governance initiatives with practical implementation strategies. Data Stewards play a vital role in executing data governance policies, enforcing data standards, and monitoring data quality metrics. Collaboration between data governance and data stewardship strengthens data management practices and fosters a culture of data responsibility within the organization.
Benefits of Strong Data Governance
Strong Data Governance yields numerous benefits for an organization, including improved data quality, enhanced decision-making capabilities, and reduced inefficiencies. By prioritizing data governance, organizations can optimize data usage, mitigate risks, and drive strategic initiatives based on reliable data insights. A robust data governance framework safeguards data assets, promotes organizational transparency, and fosters a data-driven decision-making culture.
Data Quality Management
Data quality management is a crucial aspect of the responsibilities of a data steward, especially in ensuring the accuracy and reliability of organizational data assets. In the context of this article focusing on the comprehensive guide to the responsibilities of a data steward, data quality management plays a central role in maintaining data integrity and upholding the credibility of information used for strategic decision-making. By emphasizing specific elements such as data profiling, data cleansing techniques, and monitoring data quality metrics, data quality management ensures that data remains consistent, relevant, and free from errors throughout its lifecycle.
Ensuring Data Accuracy
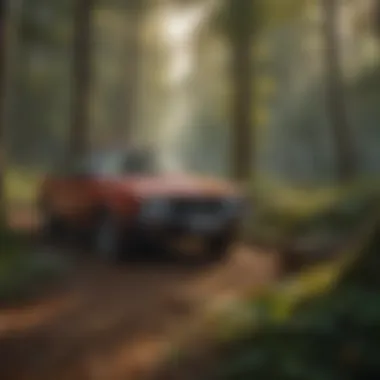
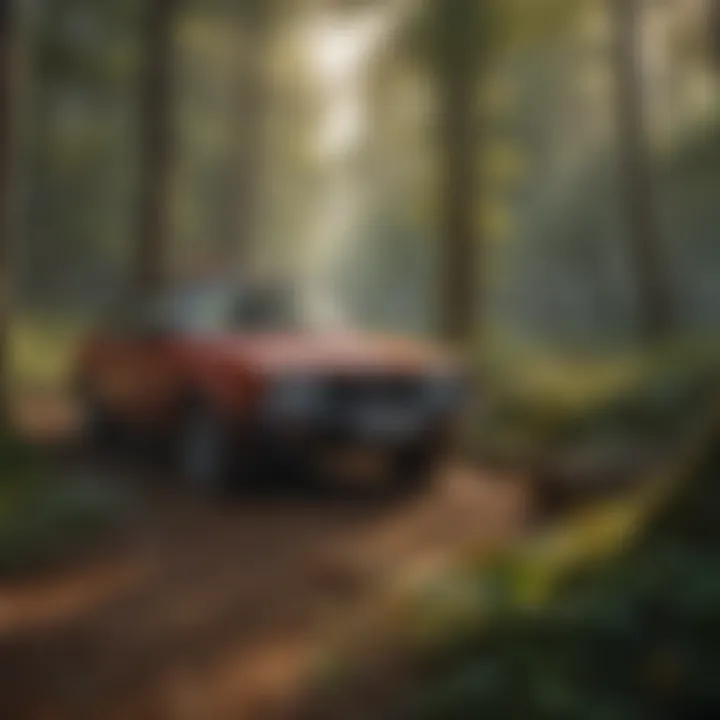
- Data Profiling
Data Profiling
Data profiling is a specialized process within data quality management that involves examining and analyzing data to understand its structure, content, and quality. In the context of this article, data profiling serves as a foundational step in ensuring data accuracy by identifying anomalies, inconsistencies, and incompleteness within datasets. The key characteristic of data profiling lies in its ability to provide data stewards with valuable insights into data quality issues, enabling them to make informed decisions on cleansing and remediation strategies. While data profiling offers extensive data visibility and understanding, challenges such as scalability and resource-intensive operations need to be considered when implementing it.
- Data Cleansing Techniques
Data Cleansing Techniques
Data cleansing techniques refer to the processes and methods used to rectify errors, inconsistencies, and redundancies within datasets, thereby improving data quality and accuracy. In the context of this article, data cleansing techniques play a vital role in enhancing the reliability and usability of data assets by cleaning, standardizing, and de-duplicating information. The key characteristic of data cleansing techniques is their ability to streamline data processing workflows and ensure that data meets predefined quality standards. While data cleansing techniques offer tangible benefits in terms of data accuracy and consistency, organizations should be mindful of potential challenges such as data loss and complexity during implementation.
- Monitoring Data Quality Metrics
Monitoring Data Quality Metrics
Monitoring data quality metrics involves the continuous assessment and evaluation of key performance indicators related to data quality, such as completeness, accuracy, timeliness, and consistency. In the context of this article, monitoring data quality metrics enables data stewards to proactively identify and address issues affecting data accuracy, integrity, and relevance. The key characteristic of monitoring data quality metrics lies in its ability to provide real-time visibility into the health of data assets and facilitate data-driven decision-making processes. While monitoring data quality metrics offers benefits such as early detection of issues and improved data governance practices, organizations need to invest in robust monitoring tools and processes to ensure effective oversight.
Implementing Data Standards
- Establishing Data Quality Rules
Establishing Data Quality Rules
Establishing data quality rules involves defining guidelines, criteria, and thresholds for acceptable data quality levels within an organization, thereby setting clear expectations and standards for data integrity and accuracy. In the context of this article, establishing data quality rules contributes to the overall goal of data quality management by enforcing consistency and compliance with data governance policies. The key characteristic of establishing data quality rules is its role in ensuring that data meets predetermined quality criteria and aligns with organizational objectives. While establishing data quality rules offers advantages such as enhanced data quality controls and decision-making processes, organizations should consider the challenges of rule maintenance and enforcement to sustain long-term effectiveness.
- Enforcing Data Quality Policies
Enforcing Data Quality Policies
Enforcing data quality policies involves implementing mechanisms, procedures, and controls to uphold data quality standards and governance requirements across the organization. In the context of this article, enforcing data quality policies serves as a cornerstone of data quality management by promoting accountability, transparency, and responsibility in data stewardship practices. The key characteristic of enforcing data quality policies is its ability to mitigate data risks, prevent breaches, and foster a culture of data-driven decision-making. While enforcing data quality policies offers benefits such as regulatory compliance and data security, organizations need to allocate resources and training to ensure consistent adherence and enforcement.
- Collaborating with Data Owners
Collaborating with Data Owners
Collaborating with data owners entails establishing partnerships, communication channels, and collaborative frameworks with stakeholders responsible for data creation, maintenance, and utilization. In the context of this article, collaborating with data owners enhances data stewardship efforts by promoting shared responsibility, knowledge exchange, and decision alignment. The key characteristic of collaborating with data owners is its capacity to bridge gaps between data governance initiatives and operational workflows, fostering a culture of data accountability and ownership. While collaborating with data owners offers advantages such as improved data trust and usability, organizations should be mindful of challenges related to data governance silos and communication barriers in fostering effective collaborations.
Data Security and Privacy
Data security and privacy stand as paramount considerations in the realm of data stewardship within organizations, playing a pivotal role in maintaining the integrity and confidentiality of sensitive data assets. In this article focusing on The Responsibilities of a Data Steward, the emphasis on data security and privacy underscores the critical importance of safeguarding information against unauthorized access, breaches, and potential vulnerabilities. By addressing specific elements such as access controls, encryption practices, and adherence to data regulations, data stewards uphold the confidentiality and integrity of data, thereby fostering trust and compliance within the organizational framework.
Safeguarding Sensitive Data
Role in Data Access Control
Delving into the specifics of data access control within the purview of data stewardship unveils its profound impact on mitigating risks of unauthorized data breaches and ensuring controlled and regulated access to sensitive information. The key characteristic of role in data access control lies in its ability to define and enforce access permissions, thereby restricting data accessibility to authorized personnel only. This article highlights the crucial significance of role in data access control as a foundational pillar in bolstering data security and privacy within organizational settings. The unique feature of role in data access control lies in its adaptability to varying data sensitivity levels and the contextual requirements of different data sets, offering a customized and scalable approach to enhancing data security measures.
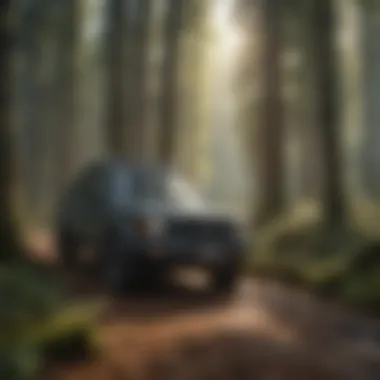
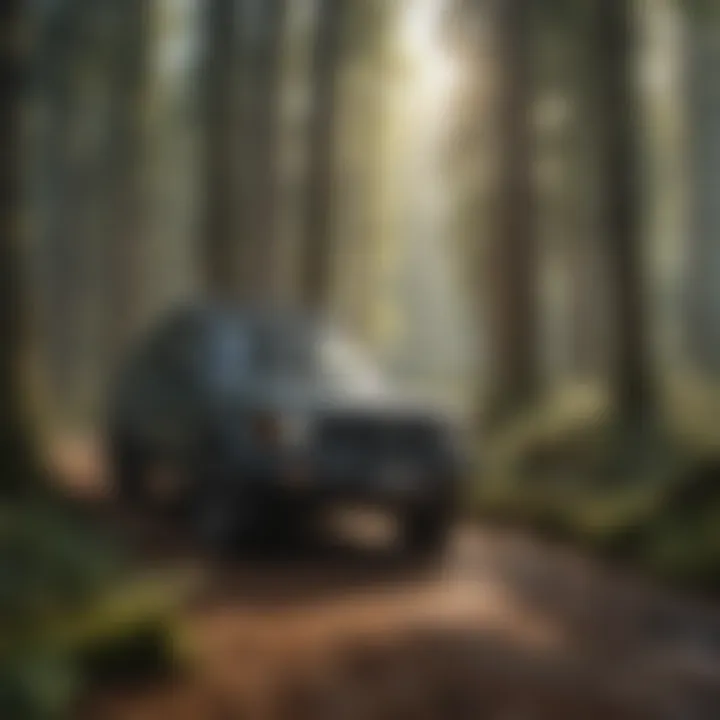
Data Encryption Practices
Data encryption practices serve as a pivotal element in fortifying data security and privacy landscapes, bringing forth encryption methodologies to protect data at rest and in transit. The key characteristic of data encryption practices centers on the transformation of plain text data into ciphertext through complex algorithms, rendering it indecipherable to unauthorized entities. This article underscores the prevalence and popularity of data encryption practices as a go-to choice for mitigating data security risks and safeguarding confidential information. The unique feature of data encryption practices lies in their ability to provide a multi-faceted approach to data protection, encompassing various encryption algorithms and key management strategies to fortify data security defenses.
Compliance with Data Regulations
Ensuring compliance with data regulations emerges as a critical aspect within the domain of data stewardship, with a profound impact on organizational data governance and risk mitigation strategies. The key characteristic of compliance with data regulations revolves around aligning data handling practices with regional, industry-specific, and organizational mandates to prevent non-compliance penalties and reputational risks. This article elucidates the mandatory nature of compliance with data regulations as a beneficial choice for fortifying data security and privacy frameworks, enhancing organizational trust and credibility. The unique feature of compliance with data regulations lies in its proactive approach to data governance, embedding regulatory requirements into day-to-day operations to uphold data integrity and protect sensitive information.
Managing Data Breaches
In the lamentable event of data breaches, data stewards are tasked with executing swift and effective incident response procedures, implementing robust data recovery strategies, and formulating proactive risk mitigation plans to address and mitigate the fallout of breaches. The comprehensive management of data breaches extends beyond mere reactive responses, encompassing proactive measures to prevent future incidents, thereby fostering a resilient and secure data environment.
Incident Response Procedures
Diving into the specifics of incident response procedures sheds light on their pivotal role in orchestrating timely and structured responses to data breaches, minimizing the impact and mitigating risks of data exposure. The key characteristic of incident response procedures lies in their systematic and agile approach to identifying, containing, eradicating, and recovering from security incidents, ensuring minimal disruption to operations. This article underscores the importance of incident response procedures as a proactive and beneficial choice for enhancing organizational readiness and resilience in the face of data breaches. The unique feature of incident response procedures lies in their adaptive nature, allowing for continuous improvement and optimization of response protocols based on evolving threat landscapes and organizational dynamics.
Data Recovery Strategies
In the aftermath of data breaches, data recovery strategies play a pivotal role in restoring data integrity, functionality, and availability to minimize operational downtime and financial losses. The key characteristic of data recovery strategies lies in their systematic approach to data restoration, encompassing backup, replication, and restoration processes to recover corrupted or lost data. This article highlights the significance of data recovery strategies as a beneficial choice for organizations to swiftly recover from data breaches and resume normal operations. The unique feature of data recovery strategies lies in their scalability and flexibility, allowing organizations to tailor recovery plans to meet specific data recovery objectives and timelines.
Risk Mitigation Planning
Proactively addressing risks and vulnerabilities through meticulous risk mitigation planning stands as a critical aspect of data stewardship in mitigating the impact of data breaches and fortifying organizational resilience. The key characteristic of risk mitigation planning lies in its proactive identification, assessment, and prioritization of risks, enabling organizations to deploy preemptive strategies to mitigate potential threats. This article emphasizes the pivotal role of risk mitigation planning as a beneficial choice for organizations to enhance cybersecurity preparedness and data breach response effectiveness. The unique feature of risk mitigation planning lies in its adaptability to dynamic risk landscapes and evolving threat scenarios, allowing organizations to stay ahead of cyber risks and formulate agile risk mitigation strategies to protect data assets.
Data Lifecycle Management
Data Lifecycle Management plays a pivotal role in this article by ensuring that data within an organization is effectively managed from acquisition to archiving. This section focuses on the specific elements, benefits, and considerations of Data Lifecycle Management.
Data Collection and Ingestion
Data Acquisition Processes
Data Acquisition Processes are a critical aspect of Data Lifecycle Management, responsible for the initial gathering of data within an organization. These processes involve the systematic collection of data from various sources, such as systems, databases, and external platforms. The key characteristic of Data Acquisition Processes is their ability to automate the retrieval of data, resulting in efficiency and accuracy in data collection. This automation makes Data Acquisition Processes a preferred choice for ensuring timely and consistent data intake. Despite their benefits, Data Acquisition Processes may face challenges in handling unstructured data formats or integrating with legacy systems, which can impact their effectiveness within this article.
Data Validation Techniques
Data Validation Techniques are essential components of Data Lifecycle Management, as they ensure the accuracy and quality of incoming data. These techniques involve the validation of data against predefined rules or standards to identify anomalies or errors. The key characteristic of Data Validation Techniques is their ability to detect inconsistencies and discrepancies in data, leading to improved data quality. By implementing Data Validation Techniques, organizations can streamline data validation processes and minimize the risk of using incorrect or incomplete data. However, the complexity of validation rules and the need for constant updating to adapt to changing data formats are challenges that organizations may encounter when using Data Validation Techniques in this context.
Integration with Data Sources
Integration with Data Sources is a crucial aspect of Data Lifecycle Management, enabling seamless connectivity between data collection points and storage systems. This integration facilitates the efficient transfer of data from source systems to designated repositories. The key characteristic of Integration with Data Sources is its ability to ensure data consistency and accessibility across different platforms. By integrating data sources, organizations can centralize their data management efforts and achieve a unified view of data assets. However, challenges such as compatibility issues between disparate systems and data silos may impact the smooth operation of Integration with Data Sources in this article.
Data Retention and Archiving
Data Retention and Archiving are fundamental components of Data Lifecycle Management, focusing on the storage and preservation of data for future reference or compliance purposes. This section delves into the specific aspects, benefits, and considerations of Data Retention and Archiving.
Defining Retention Policies
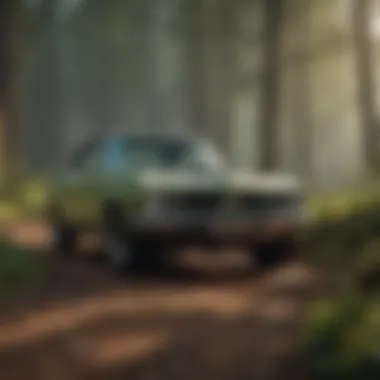
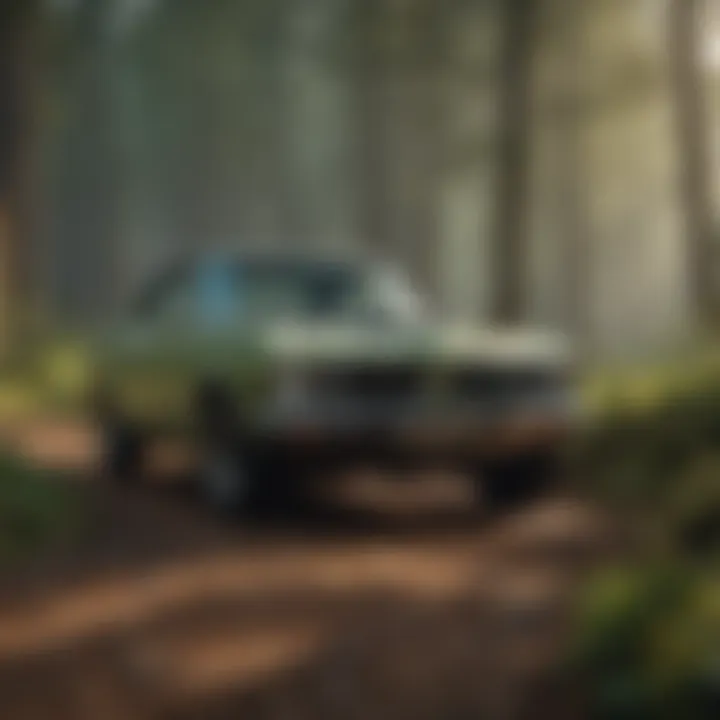
Defining Retention Policies involves establishing guidelines for determining the lifespan of data within an organization. These policies outline the duration for which data should be retained based on regulatory requirements or business needs. The key characteristic of Defining Retention Policies is their role in ensuring data compliance and minimizing storage costs. By defining clear retention policies, organizations can effectively manage data lifecycles and mitigate risks associated with prolonged data retention. However, challenges such as determining appropriate retention periods for different data types and managing data storage capacity may arise when implementing Defining Retention Policies in this article.
Archiving Strategies
Archiving Strategies encompass the methodologies and techniques used to store data in a secure and accessible manner over extended periods. These strategies involve the categorization, indexing, and storage of data in archived repositories for long-term preservation. The key characteristic of Archiving Strategies is their ability to optimize storage resources and facilitate efficient data retrieval when needed. By employing Archiving Strategies, organizations can declutter active databases and ensure data scalability for future expansion. Nevertheless, challenges such as maintaining data integrity during archiving processes and balancing access speed with storage costs can affect the implementation of Archiving Strategies within this article.
Legal Compliance Considerations
Legal Compliance Considerations address the importance of adhering to regulatory requirements and industry standards when managing data retention and archiving practices. This aspect emphasizes the need to align data storage and retention policies with relevant laws and regulations to avoid legal implications. The key characteristic of Legal Compliance Considerations is their role in safeguarding data privacy and confidentiality, thereby reducing compliance risks for organizations. By integrating legal compliance considerations into data lifecycle management, organizations can ensure data governance and mitigate legal exposure. However, challenges such as interpreting complex legal frameworks and keeping up with evolving regulatory mandates may pose hurdles in implementing Legal Compliance Considerations effectively within this article.
Collaboration and Communication
Collaboration and communication stand at the core of successful data stewardship, playing a pivotal role in ensuring effective data management. In the context of this article, collaboration refers to the harmonious interaction between various stakeholders involved in data stewardship, while communication emphasizes the exchange of vital information to facilitate seamless operations. By fostering collaboration and open communication channels, data stewards can harness collective expertise and promote a culture of transparency within organizations, thereby enhancing decision-making processes and ensuring data integrity.
Working with Cross-Functional Teams
- Engaging with Data Users
Engaging with Data Users
Engaging with data users involves active dialogue and interaction with individuals utilizing data within an organization. This aspect is crucial as it helps data stewards understand the specific needs and requirements of data users, enabling them to tailor data management strategies accordingly. The key characteristic of engaging with data users lies in its ability to bridge the gap between technical data management practices and the practical needs of end-users. By prioritizing user engagement, data stewards can enhance data usability and relevance, ultimately contributing to the overarching goals of data stewardship.
- Collaborating with IT Departments
Collaborating with IT Departments
Collaborating with IT departments entails close coordination between data stewards and technological experts to align data management practices with IT infrastructure. This collaboration is essential as it ensures the seamless integration of data governance policies and technical functionalities, leading to enhanced data security and efficiency. The key characteristic of collaborating with IT departments lies in its facilitation of smooth data operations by leveraging IT capabilities to support data stewardship objectives effectively.
- Facilitating Knowledge Sharing
Facilitating Knowledge Sharing
Facilitating knowledge sharing involves creating platforms and mechanisms to share insights, best practices, and expertise across teams involved in data stewardship. This aspect is beneficial as it cultivates a culture of continuous learning and improvement, fostering innovation and efficiency within data management processes. The unique feature of knowledge sharing lies in its ability to democratize information and promote collaboration, empowering teams to make informed decisions and drive collective success in data stewardship initiatives.
Data Governance Reporting
- Preparing Data Governance Reports
Preparing Data Governance Reports
Preparing data governance reports entails compiling and analyzing data-related information to provide stakeholders with actionable insights and recommendations. This aspect contributes to the overarching goal of data stewardship by enhancing transparency and accountability in data management practices. The key characteristic of preparing data governance reports lies in its ability to translate complex data metrics into comprehensible findings, enabling stakeholders to make informed decisions based on data-driven evidence.
- Presenting to Stakeholders
Presenting to Stakeholders
Presenting data to stakeholders involves delivering key findings and recommendations in a clear and concise manner to ensure effective decision-making. This contribution is crucial as it bridges the gap between data insights and strategic actions, guiding stakeholders towards impactful outcomes. The unique feature of presenting to stakeholders lies in its capacity to engage diverse audiences and advocate for data-driven strategies, empowering stakeholders to leverage data insights for organizational growth and sustainability.
- Driving Data-Driven Decisions
Driving Data-Driven Decisions
Driving data-driven decisions entails promoting a culture of evidence-based decision-making by utilizing data insights to inform strategic choices. This aspect is integral to data stewardship as it empowers organizations to leverage data assets effectively and drive continuous improvement. The key characteristic of driving data-driven decisions lies in its ability to pivot decision-making processes towards data-backed solutions, mitigating risks and maximizing opportunities for organizational success.